My research objectives are |
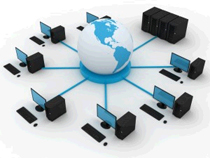 |
 |
to measure, analyze, screen, diagnose, optimize, monitor, and manage patient's mental, physical,social, and environmental conditions and contexts to maintain and improve individual and population health. |
 |
especially to timely and accurately diagnose and intervene in privacy-preserving manners, by
sensing, advanced statistics, data mining, machine learning, information security, etc. |
 |
to develop a general statistical framework for effectively and efficiently monitoring, diagnosing, and
controlling variation in data-rich environments such as the healthcare and service industries. Variability is a major factor associated with the costs of poor quality performance. Improving process predictability, stability and capability by reducing variability is the key to competitiveness. |
My research area is closely related to designing, cultivating, collecting, processing, protecting, and destructing
data over its lifecycle, which has been shown in my various accomplishment. |